Video
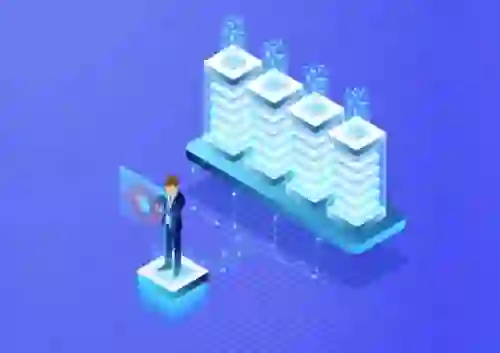
Learn more
Financial institutions are struggling to keep up with the ongoing onslaught of fraudulent transactions and cybercriminals’ changing tactics. As the global financial services landscape evolves, fraudsters are moving in tandem with the multitude of digital transaction channels, finding innovative ways to steal or fake customers’ identities and commit payment fraud.
Since the pandemic, 35% of retail banking customers increased their use of online banking. Indeed, by 2026, 53% of the world’s population is expected to use digital banking.
Online fraud has increased as digital banking usage increases, and the situation is getting worse. According to a 2022 PwC report, 51% of businesses have experienced fraud in the last two years, robbing them of an estimated $42 billion.
Banks and payment processors are working hard to detect fraud before it occurs but they have to keep up with the criminals’ evolving tactics. There’s a lot to worry about.
For some years, banks and investment companies have had to follow know-your-customer (KYC) regulations, part of which entails collecting customers’ credentials to verify their identity and statistically evaluate the risk for suspicious account activity. Standard identity verification relies on static data (for example, social security numbers don’t change, and addresses rarely do). That information isn’t updated frequently enough to be reliable or safe. The data breaches at Equifax and Capital One have shown that identity data can be stolen and used for fraud and account takeovers.
Instead, banks and financial services organizations are turning to digital identities. Document verification and biometric records are combined with intricate behavioral patterns to create a digital identity for each user. These digital identities are dynamic and complex, made up of a number of different sources and data types that are constantly changing. The challenge is updating information quickly enough to stay one step ahead of criminals without hampering the genuine user’s experience.
Financial services firms traditionally used rules-based systems to detect simple, non-changing, known fraudulent patterns such as validating black lists or user purchase profile histories. However, they do struggle to distinguish risk from normal behavior.
Machine learning (ML) algorithms and artificial intelligence (AI) predictive models can evolve and learn as they analyze and detect payment fraud based on historical and real-time transactional information. For example, transaction scoring algorithms consider transaction details, user profiles, behavioral biometrics, geolocation, IP/device metadata, a user’s financial information, and more. However, due to the size and complexity of data pipelines, successful AI/ML implementation depends not only on accurate models but also on the performance and resiliency of the underlying machine learning operations (MLOps) database, known as an online feature store.
We live in a fast-paced environment where criminals have discovered savvier ways to steal identities and credit card info to commit fraud. Financial services enterprises need to adapt to the digital age and move away from rigid and slow legacy relational database management systems (RDBMS) that cannot support modern AI/ML-based fraud detection and dynamic digital identities.
For more information, read Combat Fraud with Redis Enterprise. We go into quite a bit of detail about the ways that Redis Enterprise enables faster and more accurate fraud detection, reduces costs, and scales.