Video
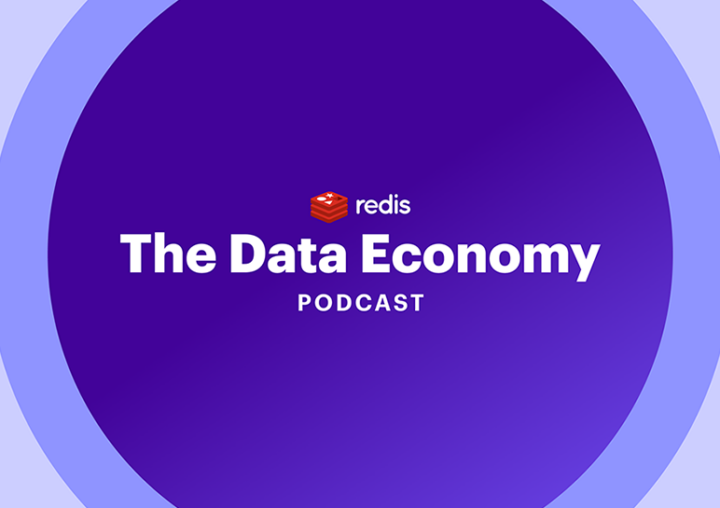
Learn more
The Data Economy is a video podcast series about leaders who use data to make positive impacts on their business, customers, and the world. To see all current episodes, explore the podcast episodes library below.
Some organizations have big data, others require real-time data processing, and a few manage the world’s most personal data. Here’s what you can learn from Experian, a company with all three data challenges.
How big is big data? How fast is real-time data processing? And how smart and innovative must data-driven organizations aspire to be when addressing business risks or pursuing new opportunities? Eric Haller, EVP & GM in Identity, Fraud & DataLabs at Experian, shares business and technical insights in the first episode of The Data Economy, a podcast presented by Redis and hosted by Michael Krigsman of CXOTalk. If you don’t know Experian, chances are they know a little bit about you. They are one of the three major U.S. credit bureaus, operates in over 40 countries, and has more than 18,000 employees.
In his role at Experian, Eric oversees the detection algorithms used to pinpoint fraudulent transactions that occur less than 1% of the time. For the majority of transactions, Experian must provide a fast response that minimizes the customer experience impact. How fast of a response? In retail, fraud detection algorithms must respond in under 200 milliseconds and, for some use cases, in under 40 milliseconds. In other words, really fast!
Eric also oversees DataLabs, Experian’s R&D team that analyzes over an exabyte of data across their four labs. One exabyte is one thousand petabytes, or 50,000 years’ worth of DVD-quality video—in other words, very big data.
And Eric reminds listeners of Experian’s data processing challenges. “We don’t want to stop a transaction unless we’re absolutely convinced there’s a significant risk, and most often, the happy path is a successful purchase,” he says.
But on the other hand, Eric acknowledges that “the data you don’t see is what usually catches you.”
So, Experian is always on the hunt for new data sources, signals to consider, machine learning features, and algorithm enhancements that improve factors such as accuracy, performance, transaction types, or geographies where it provides services.
In fact, Eric shares a story of how Experian studied the economic impact of COVID-19. In the United States, the challenge was to find the right signals that signified a local outbreak, such as a spike in people buying cough syrup. For other hotspot regions, such as Brazil, the company partnered with the United Nations, the World Health Organization, Microsoft, Amazon, and educational institutions such as the University of Chicago to aggregate new data to track virus spread. Eric conveys the cultural sentiment at Experian: “The world needs help right now, and we should do our part.”
Therein lies two lessons Eric shares in the podcast. He says, “Let the data show you how to solve the problem,” and acknowledges that Experian has significant talent, infrastructure, and experience pursuing challenges and opportunities for global and social good. For other companies, he recommends, “Be ready to work with a lot of other stakeholders that share the same dream and vision.”
It’s hard to imagine now, but it was incredibly challenging just 10 years ago to aggregate and manage extraordinarily large data sets and process super-fast, high-volume data streams for real-time decision-making. Most companies were just starting to migrate to the cloud, had few tools to centralize data, struggled with SOA implementations, and developed a lot of technology scaffolding on their own.
Today, organizations are processing time-series data, performing full-text searches on JSON documents, and are building applications with database-less architectures. Technology leaders recognize the importance of simplifying app development with a robust multi-model data platform and scaling globally while retaining local response times.
Eric shares insights on Experian’s architecture in the podcast and his perspectives on optimizing multicloud architectures, leveraging graph databases, and centralizing multi-business-unit data sources. He also discusses information security, meeting regulatory requirements, and the training Experian requires to ensure data scientists are compliant.
But with all the technology and compliance challenges, solving them is not the company’s primary objective. Eric shares his secret and says, “You would love to have the bandwidth to let your heart go wild on a data set and see what you come up with, but if you do that, you might get the one in a million needle in a haystack. You’re more likely to find winners by chasing a particular problem to solve.”
That’s great advice for developers, data scientists, and engineers, who should focus on solving the business problems and challenges first—and then seek technology partners, commercial solutions, and open source options that enable efficient technology implementations.
Isaac Sacolick, President of StarCIO, is the author of Driving Digital: The Leader’s Guide to Business Transformation through Technology which covers many practices such as agile, DevOps, and data science that are critical to successful digital transformation programs. Sacolick is a recognized top social CIO, a long-time blogger at Social, Agile, and Transformation, and a contributor at InfoWorld.
Watch more episodes of The Data Economy podcast.